Machine learning in asset pricing [electronic resource] / Stefan Nagel.
- 作者: Nagel, Stefan, 1973-
- 其他題名:
- Princeton lectures in finance.
- 出版: Princeton, NJ : Princeton University Press c2021.
- 叢書名: Princeton lectures in finance
- 主題: Capital assets pricing model. , Machine learning--Economic aspects. , Finance--Mathematical models. , Investments--Mathematical models. , Prices--Mathematical models.
- ISBN: 9780691218717 (electronic bk.) 、 0691218714 (electronic book)
- URL:
點擊此處查看電子書
點擊此處查看電子書
- 一般註:Includes bibliographical references and index. Machine learning in asset pricing -- Contents -- Preface -- Chapter 1. Introduction -- Chapter 2. Supervised Learning -- Chapter 3. Supervised Learning in Asset Pricing -- Chapter 4. ML in Cross-Sectional Asset Pricing -- Chapter 5. ML as Model of Investor Belief Formation -- Chapter 6. A Research Agenda -- Bibliography -- Index. 111年度臺灣學術電子書暨資料庫聯盟採購
-
讀者標籤:
- 系統號: 000299237 | 機讀編目格式
館藏資訊
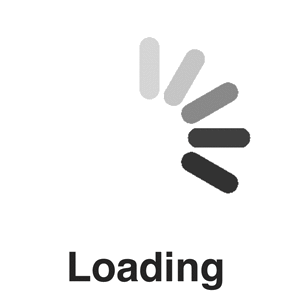
A groundbreaking, authoritative introduction to how machine learning can be applied to asset pricing Investors in financial markets are faced with an abundance of potentially value-relevant information from a wide variety of different sources. In such data-rich, high-dimensional environments, techniques from the rapidly advancing field of machine learning (ML) are well-suited for solving prediction problems. Accordingly, ML methods are quickly becoming part of the toolkit in asset pricing research and quantitative investing. In this book, Stefan Nagel examines the promises and challenges of ML applications in asset pricing. Asset pricing problems are substantially different from the settings for which ML tools were developed originally. To realize the potential of ML methods, they must be adapted for the specific conditions in asset pricing applications. Economic considerations, such as portfolio optimization, absence of near arbitrage, and investor learning can guide the selection and modification of ML tools. Beginning with a brief survey of basic supervised ML methods, Nagel then discusses the application of these techniques in empirical research in asset pricing and shows how they promise to advance the theoretical modeling of financial markets. Machine Learning in Asset Pricing presents the exciting possibilities of using cutting-edge methods in research on financial asset valuation.
摘要註
Investors in financial markets are faced with an abundance of potentially value-relevant information from a wide variety of different sources. In such data-rich, high-dimensional environments, techniques from the rapidly advancing field of machine learning (ML) are well-suited for solving prediction problems. Accordingly, ML methods are quickly becoming part of the toolkit in asset pricing research and quantitative investing. In this book, Stefan Nagel examines the promises and challenges of ML applications in asset pricing. Asset pricing problems are substantially different from the settings for which ML tools were developed originally. To realize the potential of ML methods, they must be adapted for the specific conditions in asset pricing applications. Economic considerations, such as portfolio optimization, absence of near arbitrage, and investor learning can guide the selection and modification of ML tools. Beginning with a brief survey of basic supervised ML methods, Nagel then discusses the application of these techniques in empirical research in asset pricing and shows how they promise to advance the theoretical modeling of financial markets. Machine Learning in Asset Pricing presents the exciting possibilities of using cutting-edge methods in research on financial asset valuation.