Data Preprocessing, Active Learning, and Cost Perceptive Approaches for Resolving Data Imbalance [electronic resource] / Dipti P. Rana, Rupa G. Mehta.
- 其他作者:
- 出版: Hershey, PA : IGI Global 2021
- 叢書名: Advances in data mining and database management (ADMDM) book series
- 主題: Electronic data processing--Data preparation. , Verification (Logic)--Data processing. , Data integrity. , Fake news. , Fraud investigation. , Web usage mining.
- ISBN: 9781799873730 (ebk.) 、 9781799873716 (hbk.) 、 9781799873723 (pbk.)
- URL:
點擊此處查看電子書
點擊此處查看電子書
- 一般註:"Premier reference source"--Cover. Includes bibliographical references and index. 111年度臺灣學術電子書暨資料庫聯盟採購
-
讀者標籤:
- 系統號: 000298990 | 機讀編目格式
館藏資訊
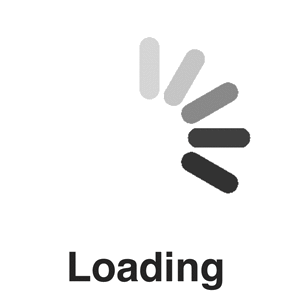
Over the last two decades, researchers are looking at imbalanced data learning as a prominent research area. Many critical real-world application areas like finance, health, network, news, online advertisement, social network media, and weather have imbalanced data, which emphasizes the research necessity for real-time implications of precise fraud/defaulter detection, rare disease/reaction prediction, network intrusion detection, fake news detection, fraud advertisement detection, cyber bullying identification, disaster events prediction, and more. Machine learning algorithms are based on the heuristic of equally-distributed balanced data and provide the biased result towards the majority data class, which is not acceptable considering imbalanced data is omnipresent in real-life scenarios and is forcing us to learn from imbalanced data for foolproof application design. Imbalanced data is multifaceted and demands a new perception using the novelty at sampling approach of data preprocessing, an active learning approach, and a cost perceptive approach to resolve data imbalance. Data Preprocessing, Active Learning, and Cost Perceptive Approaches for Resolving Data Imbalance offers new aspects for imbalanced data learning by providing the advancements of the traditional methods, with respect to big data, through case studies and research from experts in academia, engineering, and industry. The chapters provide theoretical frameworks and the latest empirical research findings that help to improve the understanding of the impact of imbalanced data and its resolving techniques based on data preprocessing, active learning, and cost perceptive approaches. This book is ideal for data scientists, data analysts, engineers, practitioners, researchers, academicians, and students looking for more information on imbalanced data characteristics and solutions using varied approaches.
摘要註
"This edited book provides a selection of chapters to improve the understanding of the impact of imbalanced data and its resolving techniques based on the Data Preprocessing, Active Learning, and Cost Perceptive Approaches"--




