Empirical approach to machine learning [electronic resource] / by Plamen P. Angelov, Xiaowei Gu.
- 作者: Angelov, Plamen P.
- 其他作者:
- 其他題名:
- Studies in computational intelligence ;
- 出版: Cham : Springer International Publishing :Imprint: Springer 2019.
- 叢書名: Studies in computational intelligence,v.800
- 主題: Engineering. , Optical pattern recognition. , Big data. , Data mining. , Computational Intelligence. , Pattern Recognition. , Big Data. , Data Mining and Knowledge Discovery. , Complexity.
- ISBN: 9783030023843 (electronic bk.) 、 9783030023836 (paper)
- URL:
點擊此處查看電子書
電子書(校內)
- 一般註:Introduction -- Part I: Theoretical Background -- Brief Introduction to Statistical Machine Learning -- Brief Introduction to Computational Intelligence -- Part II: Theoretical Fundamentals of the Proposed Approach -- Empirical Approach - Introduction -- Empirical Fuzzy Sets and Systems -- Anomaly Detection - Empirical Approach -- Data Partitioning - Empirical Approach -- Autonomous Learning Multi-Model Systems -- Transparent Deep Rule-Based Classifiers -- Part III: Applications of the Proposed Approach -- Applications of Autonomous Anomaly Detection. E1084學校採購電子書
-
讀者標籤:
- 系統號: 000274243 | 機讀編目格式
館藏資訊
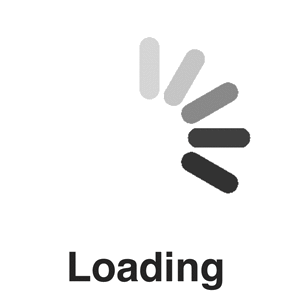
This book provides a ‘one-stop source’ for all readers who are interested in a new, empirical approach to machine learning that, unlike traditional methods, successfully addresses the demands of today’s data-driven world. After an introduction to the fundamentals, the book discusses in depth anomaly detection, data partitioning and clustering, as well as classification and predictors. It describes classifiers of zero and first order, and the new, highly efficient and transparent deep rule-based classifiers, particularly highlighting their applications to image processing. Local optimality and stability conditions for the methods presented are formally derived and stated, while the software is also provided as supplemental, open-source material. The book will greatly benefit postgraduate students, researchers and practitioners dealing with advanced data processing, applied mathematicians, software developers of agent-oriented systems, and developers of embedded and real-time systems. It can also be used as a textbook for postgraduate coursework; for this purpose, a standalone set of lecture notes and corresponding lab session notes are available on the same website as the code. Dimitar Filev, Henry Ford Technical Fellow, Ford Motor Company, USA, and Member of the National Academy of Engineering, USA: “The book Empirical Approach to Machine Learning opens new horizons to automated and efficient data processing.” Paul J. Werbos, Inventor of the back-propagation method, USA: “I owe great thanks to Professor Plamen Angelov for making this important material available to the community just as I see great practical needs for it, in the new area of making real sense of high-speed data from the brain.” Chin-Teng Lin, Distinguished Professor at University of Technology Sydney, Australia: “This new book will set up a milestone for the modern intelligent systems.” Edward Tunstel, President of IEEE Systems, Man, Cybernetics Society, USA: “Empirical Approach to Machine Learning provides an insightful and visionary boost of progress in the evolution of computational learning capabilities yielding interpretable and transparent implementations.”
摘要註
This book provides a 'one-stop source' for all readers who are interested in a new, empirical approach to machine learning that, unlike traditional methods, successfully addresses the demands of today's data-driven world. After an introduction to the fundamentals, the book discusses in depth anomaly detection, data partitioning and clustering, as well as classification and predictors. It describes classifiers of zero and first order, and the new, highly efficient and transparent deep rule-based classifiers, particularly highlighting their applications to image processing. Local optimality and stability conditions for the methods presented are formally derived and stated, while the software is also provided as supplemental, open-source material. The book will greatly benefit postgraduate students, researchers and practitioners dealing with advanced data processing, applied mathematicians, software developers of agent-oriented systems, and developers of embedded and real-time systems. It can also be used as a textbook for postgraduate coursework; for this purpose, a standalone set of lecture notes and corresponding lab session notes are available on the same website as the code. Dimitar Filev, Henry Ford Technical Fellow, Ford Motor Company, USA: "The book Empirical Approach to Machine Learning opens new horizons to automated and efficient data processing." Paul J. Werbos, Inventor of the back-propagation method, USA: "I owe great thanks to Professor Plamen Angelov for making this important material available to the community just as I see great practical needs for it, in the new area of making real sense of high-speed data from the brain." Chin-Teng Lin, Distinguished Professor at University of Technology Sydney, Australia: "This new book will set up a milestone for the modern intelligent systems." Edward Tunstel, President of IEEE Systems, Man, Cybernetics Society, USA: "Empirical Approach to Machine Learning provides an insightful and visionary boost of progress in the evolution of computational learning capabilities yielding interpretable and transparent implementations.".




